- Home
- Member Resources
- Articles
- Is it Magic? Artificial Intelligence Integration into Clinical Cytometry Workflows
Why flow cytometry lends itself to computational assistance
Cytometry-based assays are widely available and frequently used in research and clinical diagnostics. As a field, cytometry has experienced substantial innovation through the continuous development of novel reagents, new instrumentation, and improved analyses geared towards handling multi-dimensional data of up to 30 to 40 parameters.1,2 However, cytometry assays require extensive training and expertise in data acquisition and analysis. The final interpretation relies on integration of all available information, including performance characteristics of a specific assay and knowledge of “normal” cell subsets, immunophenotypes, and maturation patterns. Results can therefore be highly subjective, and direct comparisons between samples, sample types, experiments, or laboratories may be limited due to vastly different sample preparation protocols, reagents, analyzer platforms, or analysis settings and strategies.3,4
To limit subjectivity or variability and to optimize high-dimensional data representation and analysis, cytometry-based approaches therefore lend themselves to computational assistance. In addition, cytometry data can be easily fed to computational algorithms as it is by convention stored as tabular data containing experimental metadata and binary result data (Flow Cytometry Standard [FCS] format files).5 Many research settings therefore already depend greatly on computational tools and algorithms to perform batch correction, dimensionality reduction, clustering, and visualization, especially for mass cytometry and spectral flow cytometry data.6–8 By contrast, clinical diagnostic laboratories currently rarely use advanced computational tools. Common barriers are lack of computational expertise and resources, potentially lengthy computing times, and uncertainties regarding use cases, implementation, and accountability. However, several areas have emerged where artificial intelligence (AI), ie, using computational approaches to mimic human actions and thereby complete specific human tasks, could greatly facilitate the diagnostic process.9,10 Of note, AI already is being explored in several pathology settings (see prior articles on this website: https://www.cap.org/member-resources/articles/machine-learning-in-pathology-the-potential-to-predict-the-future-for-patients, https://www.cap.org/member-resources/articles/next-generation-anatomic-pathology-innovative-imaging-technologies-on-the-horizon), with substantial potential to enhance and personalize patient care. It is therefore reasonable to expect that AI integration into the clinical cytometry laboratory will have a similar impact for diagnosis, risk stratification, or outcome prediction for patients with hematologic disorders.
“The Old”: Current use of computation in clinical cytometry
Most clinical cytometry laboratories offer a range of diagnostic panels and handle large amounts of patient samples, making the entire workflow highly complex. As such, several preanalytic steps evaluating cytometer performance, system stability (flow rate) and compensation require manual inspection, and are essential to ensure assay reliability. In the analysis phase, computation-assisted workflows are geared towards depicting data in a user-friendly and easy-to-comprehend manner by applying a series of bivariate scatter plots and histograms (“gating”) and logical “if-then” associations. This approach is used for data cleaning to remove debris and doublets and to define cell populations based on cell size, granularity, and immunophenotype. The final interpretation integrates qualitative and quantitative comparisons to healthy controls, intra-sample populations, or to patterns in a patient’s previously analyzed sample. On occasion, this can require adding extra tubes to evaluate specific markers or bespoke marker combinations. This analytic process is predominantly performed in a manual fashion, making it highly operator-dependent and prone to subjectivity, especially when defining cut-off values for positivity versus negativity or marker expression levels (often describing quantitative data with qualitative terms, such as “dim”, “variable”, “small subset”, and “strong”). To limit manual gating, templates and rule-based algorithms can be employed for semi-automated gating of subpopulations using marker expression levels. While this does not eliminate the need for manual population identification, it can streamline workflows by facilitating data visualization and quantification. Batch correction to eliminate inter-sample or inter-experiment variability and downstream effects on interpretation are not commonplace in many clinical settings, although some clinically used flow cytometry software solutions provide batch analysis metrics. Together, the current approaches are labor- and time-intensive and operator-dependent. In addition, they may insufficiently resolve certain populations or immunophenotypes, especially with increasing numbers of parameters such as antigens or sample tubes, and when encountering rare or unexpected populations, thereby limiting diagnostic accuracy and discovery.
“The New”: Research applications of AI in cytometry and clinical use cases
To automate preprocessing tasks and improve data visualization and cell or sample identification, a variety of models have been developed. These are comprehensively reviewed elsewhere, and include in-depth explanations of approaches, training requirements, and extent of supervision.8–10 In brief, AI algorithms in cytometry include machine learning (ML) and deep learning (DL) approaches. ML is a subset of AI that supplies data to a computational algorithm to develop a model which can then perform defined tasks on new data. Deep learning (DL) is a type of artificial neural network-based ML modelled after the human brain that provides multiple layers of computations to accurately model complex data. Supervised models require prior labeling of samples, cells, or populations in a training dataset to teach a classifier for predicting these features in a new sample. While these models benefit from providing easily understandable outputs, performance and quality of supervised models can be restricted to data sets that are similar to the training set, hampering their generalizability. Unsupervised algorithms attempt to identify novel features or information from raw data without prior assumptions or the need for manual classification. These models may allow for greater feature discovery and flexibility but still require downstream manual interpretation.
In general, the choice of model is guided by which aspects of information in cytometry data are most important to preserve, analyze, or discover, and the desired degree of human involvement (Figure 1). Recent studies using clinical cytometry datasets have employed various supervised and unsupervised ML-/ DL-based approaches to identify clinically meaningful populations and immunophenotypes and provide sample classifications.8–11 For example, ML algorithms have proven useful to flag samples requiring additional workup based on the identification of abnormal populations or immunophenotypes.12 Moreover, several ML models been able to recognize and characterize blast populations in acute leukemia or abnormal lymphocyte populations in lymphomas, thereby providing fast, objective, and highly accurate diagnostic workups of a range of lymphomas and leukemias.13–20 ML-based algorithms have also shown enormous potential for disease progression prediction and measurable residual disease (MRD) assessments.21–24 The latter are notoriously difficult to execute and analyze, and ML-based MRD approaches have succeeded in several tasks that to date can only be inefficiently performed in a manual fashion. These included automated comparisons to other (including minor) populations, leukemia-associated immunophenotypes, patients’ prior flow cytometry results, or even large reference datasets or databases. Lastly, there is enormous potential in AI integration into preprocessing steps of the clinical workflow, for example to monitor cytometer performance (ie, fluctuations in flow rate or signal intensities), enhance or improve existing data cleaning strategies, and to perform compensation or data transformation.
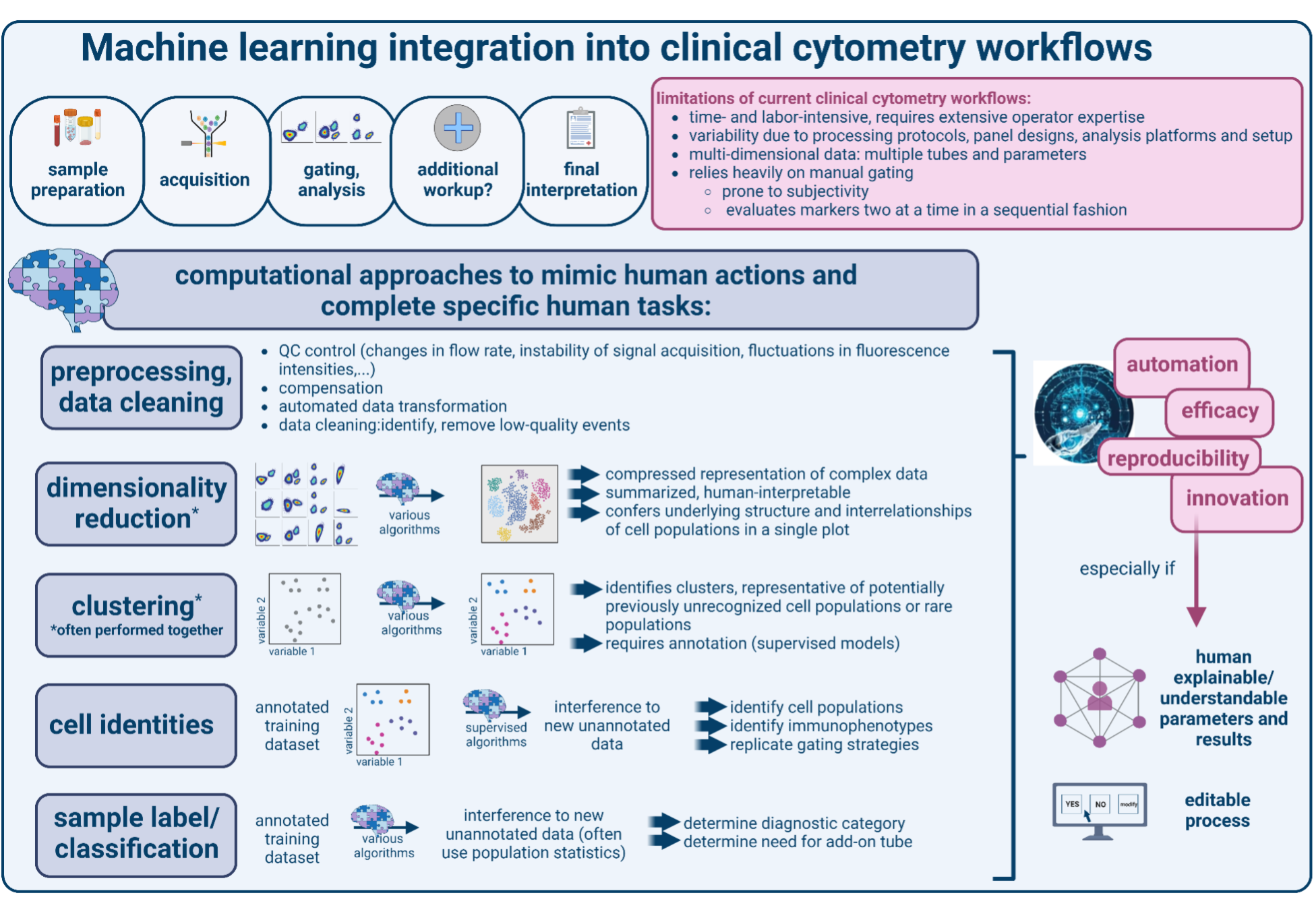
“The Undertaking”: challenges and areas of need
Overall, clinical cytometry AI algorithms have been highly accurate and greatly reduce analysis variability compared to manual approaches. This is particularly true when a combination of different algorithms is used and when algorithms have been optimized for a specific sample type or clinical use case. However, routine adoption into clinical diagnostic cytometry-based procedures faces the following challenges:
First, although flow cytometry assays are highly standardized within a specific laboratory or setting to meet accreditation requirements, there is a lack of global standardization of common flow cytometry methods and practices. This includes instrumentation, reagents and antibody panels, as well as analysis, interpretation, and reporting practices. Several recommendations have been published, but widespread adoption is pending, making direct comparisons between datasets difficult.25–27 In addition, the use of non-standardized names for protein markers, sample types, experimental conditions, and disease states is common. Information on reagents, instrumentation, panels, and diagnoses is often proprietary or not publicly available. This data diversity and lack of comparability/compatibility are particularly relevant when considering training and validating datasets for ML models, limiting their generalizability and portability. Therefore, additional research in novel cytometry-specific ML techniques that make models more robust to differences in practice is needed, and promising approaches include transfer learning and synthetic data generation.
Second, AI support of clinical decision-making and diagnostic procedures requires a thorough evaluation of clinical use cases, policies, and governance/accountability structures (Table 1). For example, the prevalence of certain diseases and the perceived risks of AI-based decisions need to be thoroughly evaluated, which informs metrics for success or failure and the definition of a clinical ground truth. In addition, local or institutional circumstances and expectations need to be considered, which may vary widely between institutions and use cases. Importantly, the discussions surrounding appropriate ethical and regulatory frameworks are ongoing, and several governmental and non-governmental organizations have issued documents requesting public feedback, blueprints, recommendations, and bills/laws/statutes. Although none of them are yet specific to clinical flow cytometry, the roles of pathologists and technical cytometry staff will likely involve ensuring fair, unbiased, and equitable outcomes by taking responsibility for data integrity, monitoring performance and stability of the model, and resolving aberrancies or inconsistencies. However, many ML models have been developed with greater emphasis on performance than interpretability. This has led to the perception of ML models as "black boxes" and amplified concerns that features used to assign abnormal or discriminative labels may not be relevant to human decision making, which might hamper their widespread adaptation.
Regardless, ongoing interdisciplinary and collaborative efforts promise to produce more reproducible and robust cytometry and ML approaches, to improve methods of knowledge transfer, to create incentives for public data sharing, to increase community and stakeholder enthusiasm, and to enhance literacy in statistical and AI methods. Eventually, this will pave the way to integrating AI-support into clinical cytometry diagnostic laboratory workflows, and in the future allow integration with other diagnostic data points, innovating biomarkers, analysis tools, and treatment strategies. After all, there is no magic in cytometry (Shapiro’s zeroth law), and AI is math – not magic!28
Table 1: Overview of clinical and governance frameworks for AI integration into clinical workflows
Factor |
Pertinent question |
Examples |
Precise characterization of requirements/ clinical use case |
What clinical problem should AI solve? |
|
Definition of metrics for success or failure |
How good does AI need to be? |
|
Agreement on the ground truth |
What counts as a correct output? |
|
Infrastructure, policies and governance |
Can AI assistance be successfully implemented and maintained? |
|
References
- Robinson JP. Flow cytometry: past and future. Biotechniques. 2022;72(4):159-169. doi:10.2144/btn-2022-0005
- Nolan JP. The evolution of spectral flow cytometry. Cytometry A. 2022;101(10):812-817. doi:10.1002/cyto.a.24566
- Czechowska K, Lannigan J, Wang L, et al. Cyt‐Geist: Current and Future Challenges in Cytometry: Reports of the CYTO 2018 Conference Workshops. Cytometry. 2019;95(6):598-644. doi:10.1002/cyto.a.23777
- Czechowska K, Lannigan J, Aghaeepour N, et al. Cyt‐Geist: Current and Future Challenges in Cytometry: Reports of the CYTO 2019 Conference Workshops. Cytometry. 2019;95(12):1236-1274. doi:10.1002/cyto.a.23941
- Spidlen J, Moore W, Parks D, et al. Data File Standard for Flow Cytometry, Version FCS 3.2. Cytometry A. 2021;99(1):100-102. doi:10.1002/cyto.a.24225
- Saeys Y, Van Gassen S, Lambrecht BN. Computational flow cytometry: helping to make sense of high-dimensional immunology data. Nat Rev Immunol. 2016;16(7):449-462. doi:10.1038/nri.2016.56
- Todorov H, Saeys Y. Computational approaches for high-throughput single-cell data analysis. FEBS J. 2019;286(8):1451-1467. doi:10.1111/febs.14613
- Hu Z, Bhattacharya S, Butte AJ. Application of Machine Learning for Cytometry Data. Front Immunol. 2021;12:787574. doi:10.3389/fimmu.2021.787574
- El Alaoui Y, Elomri A, Qaraqe M, et al. A Review of Artificial Intelligence Applications in Hematology Management: Current Practices and Future Prospects. J Med Internet Res. 2022;24(7):e36490. doi:10.2196/36490
- Fuda F, Chen M, Chen W, Cox A. Artificial intelligence in clinical multiparameter flow cytometry and mass cytometry–key tools and progress. Seminars in Diagnostic Pathology. 2023;40(2):120-128. doi:10.1053/j.semdp.2023.02.004
- Hu Z, Tang A, Singh J, Bhattacharya S, Butte AJ. A robust and interpretable end-to-end deep learning model for cytometry data. Proc Natl Acad Sci U S A. 2020;117(35):21373-21380. doi:10.1073/pnas.2003026117
- Simonson PD, Lee AY, Wu D. Potential for Process Improvement of Clinical Flow Cytometry by Incorporating Real-Time Automated Screening of Data to Expedite Addition of Antibody Panels. Am J Clin Pathol. 2022;157(3):443-450. doi:10.1093/ajcp/aqab166
- Gaidano V, Tenace V, Santoro N, et al. A Clinically Applicable Approach to the Classification of B-Cell Non-Hodgkin Lymphomas with Flow Cytometry and Machine Learning. Cancers. 2020;12(6):1684. doi:10.3390/cancers12061684
- Zhao M, Mallesh N, Höllein A, et al. Hematologist-Level Classification of Mature B-Cell Neoplasm Using Deep Learning on Multiparameter Flow Cytometry Data. Cytometry A. 2020;97(10):1073-1080. doi:10.1002/cyto.a.24159
- Ng DP, Zuromski LM. Augmented Human Intelligence and Automated Diagnosis in Flow Cytometry for Hematologic Malignancies. Am J Clin Pathol. 2021;155(4):597-605. doi:10.1093/ajcp/aqaa166
- Simonson PD, Wu Y, Wu D, Fromm JR, Lee AY. De Novo Identification and Visualization of Important Cell Populations for Classic Hodgkin Lymphoma Using Flow Cytometry and Machine Learning. Am J Clin Pathol. 2021;156(6):1092-1102. doi:10.1093/ajcp/aqab076
- Böttcher S, Engelmann R, Grigore G, et al. Expert-independent classification of mature B-cell neoplasms using standardized flow cytometry: a multicentric study. Blood Adv. 2022;6(3):976-992. doi:10.1182/bloodadvances.2021005725
- Monaghan SA, Li JL, Liu YC, et al. A Machine Learning Approach to the Classification of Acute Leukemias and Distinction From Nonneoplastic Cytopenias Using Flow Cytometry Data. Am J Clin Pathol. 2022;157(4):546-553. doi:10.1093/ajcp/aqab148
- Zhong P, Hong M, He H, et al. Diagnosis of Acute Leukemia by Multiparameter Flow Cytometry with the Assistance of Artificial Intelligence. Diagnostics (Basel). 2022;12(4):827. doi:10.3390/diagnostics12040827
- Wödlinger M, Reiter M, Weijler L, et al. Automated identification of cell populations in flow cytometry data with transformers. Comput Biol Med. 2022;144:105314. doi:10.1016/j.compbiomed.2022.105314
- Rajwa B, Wallace PK, Griffiths EA, Dundar M. Automated Assessment of Disease Progression in Acute Myeloid Leukemia by Probabilistic Analysis of Flow Cytometry Data. IEEE Trans Biomed Eng. 2017;64(5):1089-1098. doi:10.1109/TBME.2016.2590950
- Ko BS, Wang YF, Li JL, et al. Clinically validated machine learning algorithm for detecting residual diseases with multicolor flow cytometry analysis in acute myeloid leukemia and myelodysplastic syndrome. EBioMedicine. 2018;37:91-100. doi:10.1016/j.ebiom.2018.10.042
- Reiter M, Diem M, Schumich A, et al. Automated Flow Cytometric MRD Assessment in Childhood Acute B‐ Lymphoblastic Leukemia Using Supervised Machine Learning. Cytometry. 2019;95(9):966-975. doi:10.1002/cyto.a.23852
- Salama ME, Otteson GE, Camp JJ, et al. Artificial Intelligence Enhances Diagnostic Flow Cytometry Workflow in the Detection of Minimal Residual Disease of Chronic Lymphocytic Leukemia. Cancers. 2022;14(10):2537. doi:10.3390/cancers14102537
- Heuser M, Freeman SD, Ossenkoppele GJ, et al. 2021 Update on MRD in acute myeloid leukemia: a consensus document from the European LeukemiaNet MRD Working Party. Blood. 2021;138(26):2753-2767. doi:10.1182/blood.2021013626
- Tettero JM, Freeman S, Buecklein V, et al. Technical Aspects of Flow Cytometry-based Measurable Residual Disease Quantification in Acute Myeloid Leukemia: Experience of the European LeukemiaNet MRD Working Party. HemaSphere. 2022;6(1):e676. doi:10.1097/HS9.0000000000000676
- CLSI. Validation of Assays Performed by Flow Cytometry. 1st ed. CLSI Guideline H62. Clinical and Laboratory Standards Institute; 2021.
- Shapiro H. Practical Flow Cytometry | Wiley Online Books. 3rd ed. John Wiley & Sons, Inc.; 2003. doi:10.1002/0471722731
Acknowledgements
Figures created with BioRender.com
Joshua Lewis, MD, PhD, is a Clinical clinical pathology resident at Brigham and Women's Hospital, Boston, MA, with an interest in machine learning applications to enhance hematopathology diagnostics.
Chung-Che (Jeff) Chang, MD, PhD, FCAP, is the medical director of Hematology and Molecular/Genomic Laboratory at AdventHealth-Orlando, and professor of pathology, University of Central Florida. He currently serves as associate editor for Archives of Pathology and Laboratory Medicine, and a member of the Personalized Healthcare Committee for the College of American Pathologists (CAP). He was the principal investigator of several National Institutes of Health (NIH)/National Cancer Institute (NCI) grants to study myeloma, myelodysplastic syndromes, and lymphoma. Dr. Chang’s research interests include the use of next-generation sequencing technologies for clinical diagnostics and biomarker discovery.
Fabienne Lucas, MD, PhD, is an assistant professor at the University of Washington’s School of Medicine, Department of Laboratory Medicine and Pathology, Division of hematopathology, and associate director of hematology/ coagulation. She is an International Society for Advancement of Cytometry (ISAC) Marylou Ingram scholar, and currently serves as Member-J of the CAP PHC Committee. Dr. Lucas has a research interest in AI-based clinical decision support tools in hematology and hematopathology diagnostics.